Data integrity stands as a paramount concern for regulators globally. It is due to poor practices develop by the industry. Collection of various types of information and results collectively called as data. This data are the most valuable assets of any organization but without integrity, this data is not much useful. Accuracy and original data surge the chances of stability and performance of an organization.
Data integrity refers to the degree to which all data maintain completeness, consistency, and accuracy throughout their lifecycle. Integral to this concept is the implementation of Good Documentation Practice, which safeguards data against alterations, duplications, or transfers. In data integrity, all type of data like-raw data, metadata which may be recorded both in paper or electronic form.
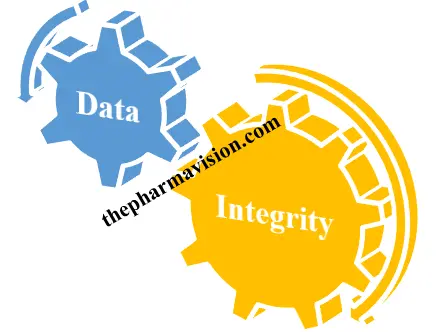
As per MHRA, GMP data integrity for industry March 2015. Data Integrity is defined as “the extent to which all data are complete, consistent and accurate, throughout the data lifecycle”.
Before a pharmaceutical product available for a patient, the manufacturing company has to present evidence of efficacy and safety. ALCOA in pharmaceuticals is used to ensure that the quality of the evidence collected is maintained as per regulatory guidelines. To safeguard data integrity, regulatory bodies such as the FDA, Health Canada and the EMEA recommend the implementation of ALCOA principles to uphold good documentation practices in pharmaceuticals.
ALCOA Principles:
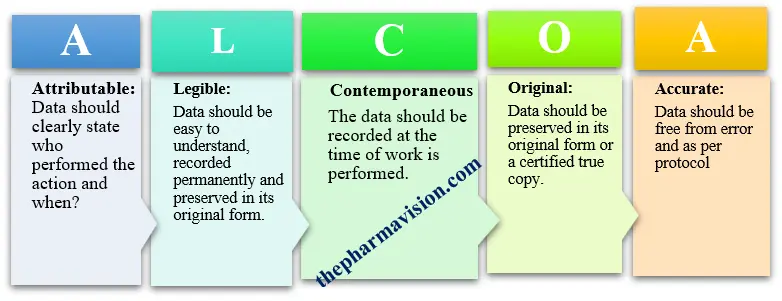
Attributable
Legible:
Contemporaneous:
Original:
Accurate:
ALCOA Plus:
Complete:
Consistent:
Enduring:
Available:
Data:
Archival:
Raw Data:
As per 21 CFR (Code of Federal Regulations) regulations, “raw data” refers to the original records and documentation that are generated during the conduct of a regulated activity, such as the manufacturing, testing, or distribution of drugs, medical devices, or other regulated products. Raw data includes all source data, metadata, and any subsequent transformations or reports derived from the original records.
Raw data must be recorded contemporaneously and accurately by permanent bases. For certain basic electronic instruments like balances or pH meters that do not store electronic data or only offer printed outputs, the printout is considered as the raw data.
Meta Data:
Metadata is data that provides context and meaning by describing the attributes of other data. Metadata describes various attributes of the primary data, such as its structure, format, location, source, creation date, authorship, and usage. It serves to provide context and facilitate the understanding, management, and use of the primary data.
Examples of metadata include:
1. File metadata: Information about a file stored on a computer system, such as its file type, size, creation date, and last modified date.
2. Document metadata: Information embedded within a document, such as the title, author, keywords, and subject.
3. Database metadata: Information about the structure and organization of a database, including table names, column names, data types, and relationships between tables.
4. Website metadata: Information embedded in a web page’s HTML code, such as the page title, description, and keywords, which help search engines index and display the page in search results.
5. Consider the number 8 in a weighing context. Without metadata like the unit (e.g., mg), its value lacks significance. Similarly, metadata provides crucial details such as the time/date stamp of the activity, the operator ID, instrument ID, processing parameters, sequence files, audit trails, and other contextual information essential for comprehending data and reconstructing activities.
Metadata plays a crucial role in data management, data governance, and data discovery processes. It enables efficient searching, retrieval, and analysis of data, as well as ensuring data quality, integrity, and security.
Static Data:
A static record format is a fixed data document (e.g., paper record or an electronic image), It is one that is fixed and allows no or very limited interaction between the user and the record content. For example, once printed or converted to static pdf, chromatography records lose the capabilities of being reprocessed or enabling more detailed viewing of baselines or any hidden fields.
Dynamic data:
Dynamic data refers to information that is subject to change or update over time. Unlike static data, which remains constant, dynamic data is characterized by its variability and ability to be modified or refreshed regularly.
Examples of dynamic data include:
1. Real-time sensor readings: Measurements or observations collected continuously from sensors or monitoring devices, such as temperature readings, stock prices, or weather data.
2. Transactional data: Records of business transactions, such as sales orders, invoices, or financial transactions, which are added, modified, or deleted as transactions occur.
3. User-generated content: Data generated by users in online platforms, such as social media posts, comments, or reviews, which can be added or edited over time.
4. System logs: Records of system activities, events, or errors generated by software applications or network devices, which are continuously updated as new events occur.
Dynamic data plays a vital role in providing up-to-date information, supporting real-time decision-making, and enabling dynamic interactions within systems, applications, and digital environments. It requires robust mechanisms for capturing, processing, and managing changes effectively to ensure data accuracy, consistency, and integrity.
Electronic data:
Electronic data refers to information that is stored, transmitted, or processed in digital form using electronic devices or systems. This includes any data that is created, collected, manipulated, or stored electronically, without the need for physical, tangible storage media such as paper. This includes data derived from ERP software utilized in quality system management, electronic laboratory data, and various records.
Quality Risk Management (QRM):
This refers to a systematic process for the assessment, control, communication and review of risks to the quality of the drug (medicinal) product across the product life cycle.
Data Life Cycle:
The data lifecycle encompasses the phases that data undergoes, starting from its creation or acquisition to its eventual deletion or archival. It encompasses the processes of data creation, storage, usage, sharing, archiving, and deletion, as well as associated activities such as data processing, analysis, and management. Throughout the data lifecycle, organizations must implement appropriate measures for data governance, security, quality assurance, and compliance to ensure the integrity, confidentiality, and availability of data. Effective management of the data lifecycle helps optimize data utilization, mitigate risks, and meet organizational objectives and regulatory obligations.
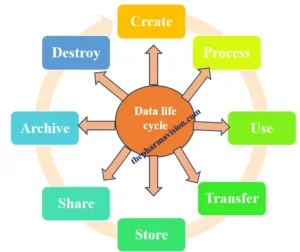
True Copy:
True copy is an exact verified copy of an original record (e.g. analytical summary reports, validation reports etc.) of data. That has been certified to confirm it is an exact and complete copy that preserves the entire content and meaning of the original record. These data must be controlled during their life cycle to ensure that the data received from another site (sister company, contractor etc.) are maintained as “true copies”.
Importance of Data Integrity:
Data in its final state is the driving force behind industry decision making. Raw data must be changed and processed to reach a more usable format. Data integrity ensures that the data is attributable, legible, contemporaneous, original, and accurate (ALCOA). Maintaining data integrity is a necessary part of the industry’s responsibility to ensure the safety, effectiveness, and quality of their products. Regulatory bodies increased attention to data integrity since several years, the FDA and various international regulatory agencies have underscored the significance of precise and dependable data to ensure the safety and quality of pharmaceuticals.
World Regulatory Guidance on Data Integrity:
USFDA: 21-CFR:
21-CFR (Code of Federal Regulation) is a codification of the general and permanent rules published in the federal register by the executive departments and agencies of the Federal Government. Title 21 of the Code of Federal Regulations (CFR) pertains to regulations established by the Food and Drug Administration (FDA). Each title or volume of the CFR undergoes revision once annually, typically around April 1st of each calendar year.
MHRA:
The MHRA guidance on GMP data integrity expectations for the pharmaceutical industry is designed to supplement the existing EU GMP guidelines concerning active substances and dosage forms. Data integrity is essential within the pharmaceutical quality system, ensuring that medicines meet the necessary quality standards.
TGA:
Australian regulatory body Therapeutic Goods Administration (TGA) give the requirement of data integrity in the form of deficiency. A deficiency in a procedure or operation that has led to, or may lead to, a substantial risk of manufacturing a product that poses harm to the consumer. This also encompasses situations where fraudulent activities, misrepresentation, or falsification of products or data by the manufacturer are observed.
cGMP:
As a reflection of the importance of this issue FDA released guidance on Data Integrity and Compliance with cGMP within the guidance itself the FDA notes the trend of increasing data integrity violations. Following cGMP-compliant record-keeping practices safeguards against the loss or obfuscation of data. FDA’s authority for cGMP comes from FD&C Act section 501 a drug shall be deemed adulterated if “the methods used in, or the facilities or controls used for, its manufacture, processing, packing, or holding do not conform to or are not operated or administered in conformity with current good manufacturing practice to assure that such drug meets the requirement of the act as to safety and has the identity and strength, and meets the quality and purity characteristics, which it purports or is represented to possess”.
Good Documentation Practices:
Within the framework of these guidelines, good documentation practices covers measures that guarantee documentation whether in paper or electronic form is attributable, legible, traceable, permanent, contemporaneously recorded, original, and accurate, both collectively and individually.
WHO:
WHO has introduced data integrity guidelines aimed at safeguarding patients globally in the realm of essential medicines and health products. These guidelines propose international good practices for regulatory authorities and inspectors to mitigate instances of incomplete data presentation or deliberate falsification by manufacturers. A crucial aspect involves ensuring the robustness and accuracy of data submitted by manufacturers to national regulatory authorities. Such data must be comprehensive, complete, and faithfully represent the quality of studies supporting applications for the introduction of medicines to the market. Additionally, adherence to standards such as good manufacturing practices (GMP), good clinical practice (GCP), and good laboratory practices (GLP) is imperative.
EME:
The European Medicines Agency (EMA) has issued updated Good Manufacturing Practice (GMP) guidance aimed at upholding the integrity of data generated during the testing, manufacturing, packaging, distribution, and monitoring of medicines. Regulators depend on these data to assess the quality, safety, and efficacy of medicines and to monitor their benefit-risk profile over time. Effective control of data records is vital to ensuring the accuracy and consistency of generated data, thereby facilitating informed decision-making by pharmaceutical manufacturers and regulatory authorities.
Management Responsibility:
It is frequently observed that management using a “Rule by Fear” approach with employees, where employees simply comply with orders and results in a culture of fear and blame, discouraging employees from challenging or adhering to regulatory guidelines.
a. Insufficient education can lead to poor decision-making or inappropriate behavior, stemming from an understanding of “How” without comprehending the underlying “Why.” Complex systems or poorly designed systems may inadvertently promote and sometimes enforce unethical practices.
b. Employees should be encouraged to utilize an open-door policy to reach top management within the organization to address compliance issues and discuss potential concerns regarding data reliability.
Common Data Integrity Issues:
User privileges:
The software system lacks adequate user level definitions and segregation, leading to inappropriate access privileges. Users may have access to functionalities such as method modification and integration, which they shouldn’t possess.
Common passwords:
Sharing of passwords among analysts obscures accountability for record creation or modification, thus compromising the ‘A’ in ALCOA (attributable, legible, contemporaneous, original, and accurate).
Computer system control:
Laboratories fail to implement sufficient controls over data, allowing unauthorized access to modify, delete, or manipulate electronic files. Consequently, the integrity of these files—originality, accuracy, and completeness—cannot be guaranteed.
Audit Trail capture:
It is recommended by the FDA that audit trails, which document changes to critical data, undergo review with each record and before final approval. These audit trails should encompass changes to crucial parameters such as finished product test results, sample run sequences, sample identification, and critical process parameters. Specific events to be monitored include overwriting, aborted runs, testing to achieve compliance, data deletion, backdating, and data alteration.
The reason of issue:
There is various reason for data integrity issue some of them write the following:
1. No raw data to support records or loss of data during changes to the system
2. Creating inaccurate and incomplete records
3.The use of test results from one batch to authorize the release of subsequent batches.
4. Backdating
5. Discarding data repeated tests, trial runs, sample runs (testing into compliance)
6. Changing the integration parameters of chromatography data to achieve acceptable results.
7. Modifying or erasing electronic records, or creating falsified data.
8. Turning off audit trail
9. Sharing password
10. Inadequate controls for access privileges
11. Inadequate/incomplete computer validation.
12. Activities not recorded contemporaneously
13. Employees that sign that they completed manufacturing steps when the employees were not on premises at the time the steps were completed
References:
1. MHRA. GMP Data Integrity Definitions and Guidance for Industry Revision, 2015.
2. Guidance on good data and record management practices, WHO Technical Report Series. 2016; 5:165-209
3. Stephen Hart, Data Integrity TGA Expectations, PDA conference, 2015.